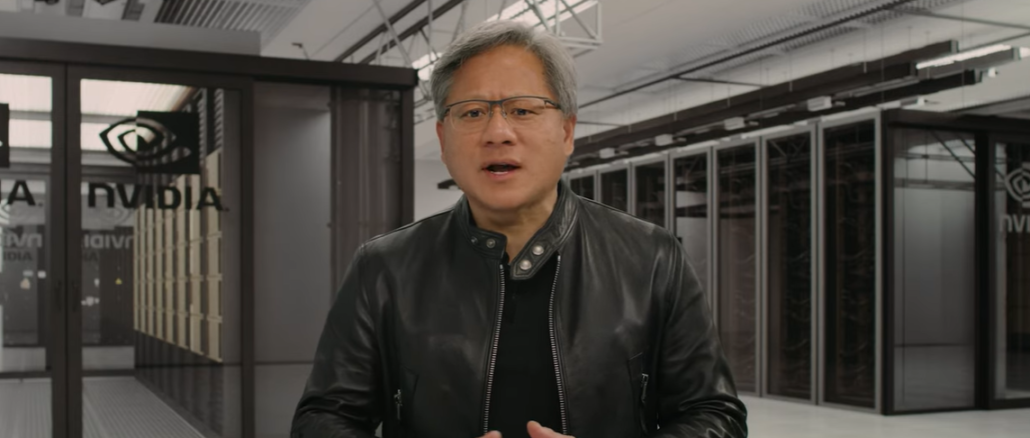
For a decade and a half, Nvidia has come a long way from its early supplier of graphics chips for personal computers and other consumer devices.
Jenson Huang, co-founder of Nvidia and CEO, turned to the data center, pressing GPUs as a way to accelerate HPC applications and the CUDA software development environment as a way to make that happen. Five years later, Huang said AI as the future of computers and that Nvidia will not only allow this, but will bet the company that this is the future of software development. Anything improved with artificial intelligence would actually be the next platform.
The company continued to grow, with Nvidia expanding its hardware and software capabilities to meet the demands of the ever-changing IT landscape, which now includes multiple clouds and fast-growing countries and, Huang expects. virtual world of digital twins and avatars and it all depends on the company’s technology.
Nvidia has not been a supplier of point products for some time, but now it is full stack platform provider for this new computer world.
“Accelerated computing begins with Nvidia CUDA general-purpose programmable GPUs,” Huang said during his keynote address at the GTC 2021 virtual event this week. “The magic of accelerated computing comes from the combination of CUDA, the acceleration libraries of algorithms that accelerate applications, and the distributed computing systems and software that scale processing across an entire data center.”
Nvidia has been perfecting CUDA and expanding the surrounding ecosystem for more than fifteen years.
“We optimize across the stack by constantly iterating between GPUs, acceleration libraries, systems and applications, constantly expanding the scope of our platform by adding new domains to the applications we are accelerating,” he said. “With our approach, end users experience accelerations throughout the life of the product. It is not uncommon for us to increase application performance with many X-factors on the same chip over several years. As we accelerate more applications, our partner network sees growing demand for Nvidia platforms. Starting with computer graphics, the scope of our architecture has reached deep into the largest industries in the world. We start with amazing chips, but for every field of science, industry and application we create a full stack. ”
To illustrate this, Huang listed more than 150 software development kits targeting a wide range of industries, from design to life sciences, and the GTC announced 65 new or updated SDKs covering areas such as quantum calculation, cybersecurity and robotics. The number of developers using Nvidia’s technology has grown to nearly 3 million, a sixfold increase over the past five years. In addition, CUDA has been downloaded 30 million times in 15 years, including 7 million times last year.
“Our experience in accelerating the full stack and data center-scale architectures allows us to help researchers and developers solve problems on the largest scale,” he said. “Our approach to calculations is highly energy efficient. The versatility of architecture allows us to contribute to fields ranging from AI through quantum physics to digital biology and climate science.
However, Nvidia is not without its challenges. The company $ 40 billion offer for Arm it is not certain, as regulators in the UK and Europe say they want to look more closely at the potential market impacts the deal will create, and Qualcomm is the leading opposition to the proposed acquisition. In addition, competition in GPU-accelerated computing is heating up, with AMD improving its capabilities – we recently wrote about Aldebaran Instinct MI200 GPU Accelerator – and Intel said last week that it expects the upcoming Aurora supercomputer to do so scale over 2 exaflops largely due to the better-than-expected performance of his “Ponte Vecchio” Xe HPC graphics processors.
However, Nvidia sees its future in laying the groundwork for accelerated computing to expand AI, machine learning, and in-depth training across a wide range of industries, as illustrated by the usual avalanche of messages coming from the GTC. Among the new libraries was ReOpt, which aims to find the shortest and most efficient routes to deliver products and services to their destinations, which can save companies time and money in last mile delivery efforts.
CuQuantum is another library for creating quantum simulators to validate research in the field as the industry builds the first useful quantum computers. Nvidia has built a cuQuantum DGX device to accelerate quantum circuit simulations, with the first accelerated quantum simulator coming in Google’s Cirq framework, coming in the first quarter of 2022. Meanwhile, cuNumeric aims to accelerate NumPy workloads, scalable from a single GPU. many round-the-clock clusters.
Nvidia’s new Quantum-2 connection (which has nothing to do with quantum computing) is a 400 Gb / sec InfiniBand platform that includes the Quantum-2 switch, ConnectX-7 SmartNIC, BlueField 3 DPU, and features such as telemetry-based performance isolation. congestion control system and 32X higher processing in AI training switch. In addition, nanosecond synchronization will allow cloud data centers to enter the telecom space by hosting software-defined 5G radio services.
“Quantum-2 is the first network platform to offer supercomputer performance and the ability to share cloud computing,” Huang said. “It has never been possible before. Up to Quantum-2 you get either bare metals with high performance, or secure multi-hire. Never both. With Quantum-2, your valuable supercomputer will be clouded and used much better. ”
The 7-nanometer InfiniBand switch chip contains 57 billion transistors – similar to Nvidia’s A100 GPU – and has 64 ports running at 400 Gb / sec or 128 ports running at 200 Gb / sec. The Quantum-2 system can connect up to 2048 ports, compared to 800 ports with Quantum-1. The switch is being tested now and comes with options for the ConnectX-7 SmartNIC – sampling in January – or the BlueField 3 DPU, which will be tested in May.
BlueField DOCA 1.2 is a set of cybersecurity capabilities that Huang says will make BlueField an even more attractive platform for building a zero-confidence architecture by unloading infrastructure software that consumes up to 30% of CPU capacity. In addition, Nvidia’s Cybersecurity Morpheus deep learning platform uses AI to monitor and analyze data from users, machines, and services to detect anomalies and abnormal transactions.
“Cloud computing and machine learning are leading to a rediscovery of the data center,” Huang said. “Container-based applications give hyperscalers incredible scaling capabilities, allowing millions to use their services simultaneously. Ease of scaling and orchestration comes at a price: East-West network traffic has increased tremendously with machine-to-machine messaging, and these disaggregated applications open many ports in the data center that need to be protected from cyberattacks.
Nvidia has strengthened its Triton Inferencing Server with new support for the Arm architecture; the system already supports Nvida GPU and X86 chips from Intel and AMD. In addition, Triton version 2.15 can also run multiple GPUs and multi-node workloads, which Huang called “one of the most technically challenging performance engines the world has ever seen.”
“As these models grow exponentially, especially in new applications, they often become too large to run on a single processor or even a single server,” said Ian Buck, vice president and general manager of data center business. Nvidia said during a press briefing. “And yet the demands [and] the capabilities for these large models want to be provided in real time. The new version of Triton actually supports distributed inference. We take the model and divide it into multiple GPUs and multiple servers to deliver it, to optimize the calculations to ensure the fastest possible performance of these incredibly large models.
Nvidia also introduced the NeMo Megatron, a large language model (LLM) learning framework that has trillions of parameters. NeMo Megatron can be used for such tasks as language translation and writing computer programs and uses Triton Inference Server. Nvidia last month introduced the Megatron 530B, a language mode with 530 billion parameters.
“The recent breakthrough of major language models is one of the great advances in computer science,” Huang said. “There is exciting work being done in self-controlled multimodal learning and models that can perform tasks that have never been taught – called zero-chance learning. Ten new models were announced last year alone. LLM training is not for the faint of heart. One hundred million dollar systems, training models with trillions of parameters on petabytes of data for months requires conviction, deep experience and an optimized stack.
Much of the event was spent on Nvidia’s Omniverse platform, a virtual environment introduced last year that the company believes will be a critical enterprise tool in the future. Skeptics point to avatars and the like, suggesting that the Omniverse is little more than a second coming of Second Life. In response to a question, Buck said there are two areas in which Omniverse captures the company’s success.
The first is digital twins, virtual representations of machines or systems that recreate “an environment like the work we do in embedded and robotics and other places so that we can simulate virtual worlds, in fact simulate products that are built in a virtual environment. environment and be able to prototype them entirely with Omniverse. The virtual setup allows product development to take place in a way that was previously remote, virtually worldwide.
The other is in the commercial use of virtual agents – this is the place AI based avatars can come in – to help with call centers and similar tasks aimed at customers.